Understanding Labelling Tool Machine Learning: The Future of Data Annotation
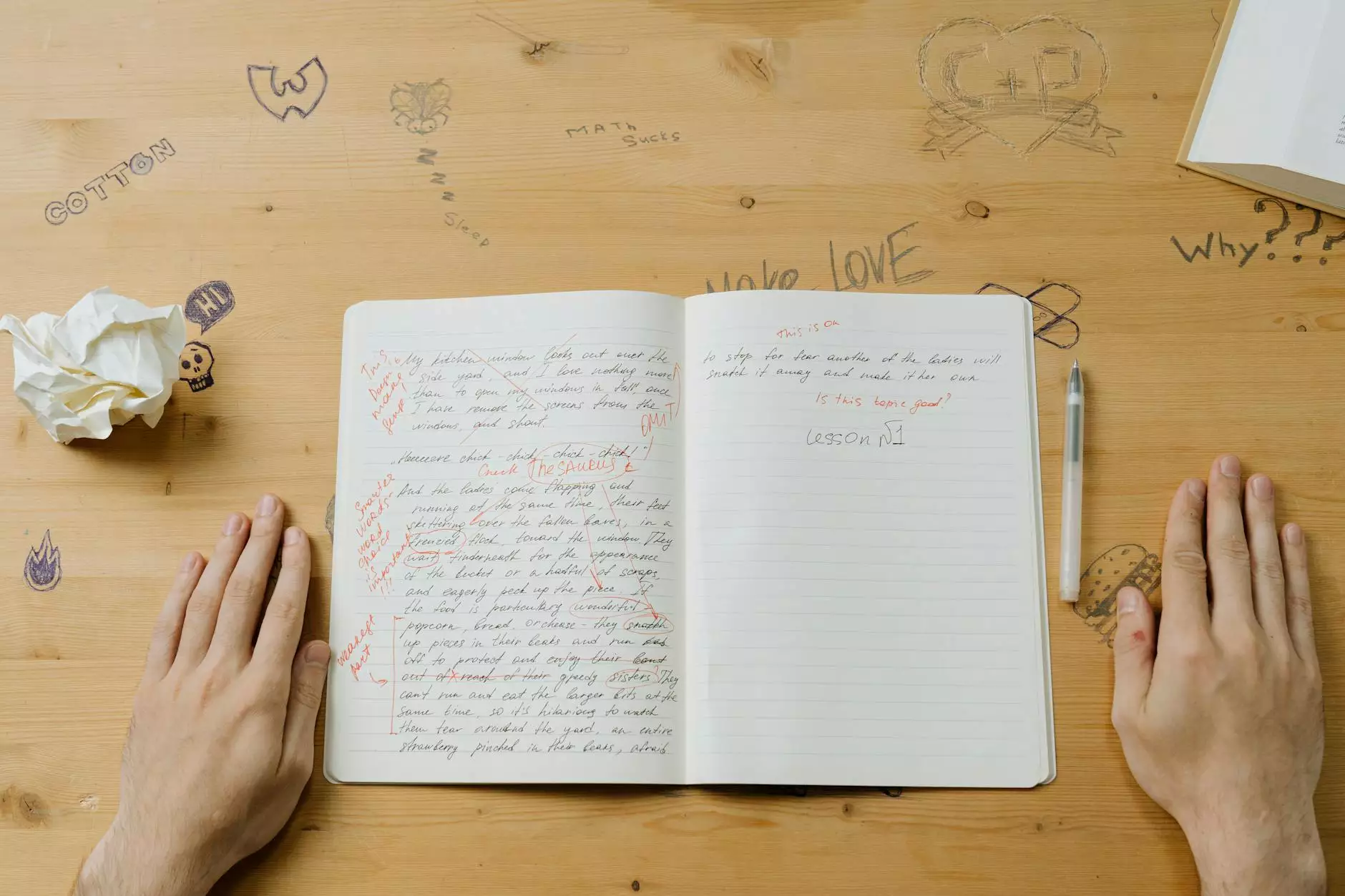
Labelling tool machine learning is transforming the landscape of how businesses utilize data. In the age of information, data serves as the backbone of innovative solutions and intelligent decision-making. Companies are increasingly realizing the immense value embedded in their data, leading to a surge in demand for effective data annotation platforms and tools. This article delves into the complexities and advantages of labelling tools and illustrates their pivotal role in bolstering machine learning endeavors.
What is Data Annotation?
Data annotation is the process of labeling data for machine learning models to understand, interpret, and make predictions based on that data. This process is essential for the training of various AI systems, significantly impacting the effectiveness and accuracy of these models. Here’s a more detailed analysis of what data annotation entails:
- Types of Data Annotation: Data can be annotated in various forms including text, audio, image, and video data. Each type has unique considerations and challenges.
- Importance of Quality: Quality in data annotation directly influences the performance of machine learning models. Properly labelled data ensures that models can learn patterns effectively.
- Human vs. Automated Annotation: While human annotators can provide nuanced understanding, automated labelling tools leverage machine learning to speed up the process and reduce costs.
The Role of Labelling Tools in Machine Learning
Labelling tools serve as the infrastructure that supports the data annotation process. They streamline the task of assigning labels to datasets, ensuring that machine learning algorithms receive the highest quality inputs. The functionalities of these tools encompass:
Efficiency and Speed
By integrating a labelling tool machine learning framework, businesses can exponentially increase the speed at which they annotate data. This efficiency means faster model development cycles and shorter time-to-market for AI solutions.
Scalability
As the volume of data continues to grow, companies require labelling solutions that can scale accordingly. Modern labelling tools are designed to handle a vast array of datasets and can easily adapt to larger projects without sacrificing quality.
Collaboration and Accessibility
Many labelling tools enable collaborative functionalities, allowing teams to work together on data annotation projects. This feature is critical for organizations where cross-departmental collaboration is necessary for comprehensive data coverage.
Key Features of Effective Labelling Tools
When considering a labelling tool, several features are essential to ensure satisfactory results:
- User-Friendly Interface: A tool with an intuitive interface can facilitate onboarding and usage by non-technical staff.
- Customizability: Businesses often have unique needs; hence, the ability to customize labels, actions, and workflows is crucial.
- Quality Assurance Protocols: Implementing features such as review systems and feedback loops ensures data quality and reliability.
- Integration with Machine Learning Pipelines: It's essential for labelling tools to seamlessly integrate with existing machine learning frameworks and models.
The Business Impact of Machine Learning Labelling Tools
Leveraging strong labelling tools has profound implications for businesses. Here are some of the critical advantages:
Enhanced Decision Making
With accurately annotated data, businesses are empowered to make more informed decisions. Whether it's predicting consumer behavior or optimizing supply chains, quality data leads to quality insights.
Cost Efficiency
Automated labelling tools can significantly reduce the costs associated with manual data annotation. This reduction means organizations can invest those resources elsewhere, maximizing ROI.
Improved Product Offerings
Product development greatly benefits from insights drawn from well-annotated data. Companies can innovate effectively, using real user data to drive enhancements and new features.
Challenges in Data Annotation and Machine Learning
While labelling tools offer significant advantages, challenges remain in data annotation:
Quality Control
Ensuring high quality in annotated datasets is crucial. Mistakes in labelling can propagate through machine learning models, leading to inaccurate predictions and outcomes.
Data Privacy Concerns
As organizations collect and annotate vast amounts of data, ensuring data privacy and compliance with regulations such as GDPR becomes a significant challenge.
Handling Subjectivity in Annotation
Different annotators may interpret data in various ways, leading to inconsistencies. It's important to have clear guidelines and training for annotators to minimize subjectivity.
Future Trends in Labelling Tools for Machine Learning
As the use of machine learning continues to expand, so do labelling tools and their capabilities. Here are some enlightening trends to watch:
AI-Assisted Annotation
AI technologies are starting to offer preliminary labelling suggestions, helping humans complete tasks faster. This hybrid approach enhances productivity while maintaining quality.
Integration with No-Code Platforms
As no-code platforms gain traction, integrating labelling tools with these platforms will democratize access to powerful data processing capabilities, allowing non-technical users to contribute meaningfully.
Real-time Collaboration Features
The need for teams to collaborate in real-time on data projects continues to grow, spurring the development of innovative features in labelling tools aimed at fostering effective team dynamics.
Conclusion: Embracing the Power of Labelling Tools in Machine Learning
The advent of labelling tool machine learning signifies a leap forward in how data is processed and utilized across industries. By investing in effective labelling solutions, organizations can harness their datasets' full potential, enabling smarter decisions, enhancing operational efficiency, and paving the way for innovative products and services.
As the business landscape evolves with rapid technological advancements, the need for high-quality annotated data remains paramount. Companies must stay ahead of the curve by adopting state-of-the-art labelling tools to ensure they remain competitive in an increasingly data-driven world. Key Labs AI is at the forefront of this evolution, offering cutting-edge data annotation tools and platforms that transform raw data into actionable insights, driving innovation and growth.
Get Started with Key Labs AI
If your organization is looking to enhance its machine learning capabilities through superior data annotation, Key Labs AI provides the resources and expertise you need. Explore our Data Annotation Tool and Data Annotation Platform to unlock the true potential of your data.